Three Keys to Substantial Compliance in Cross-Border Mergers and Acquisitions
Three Keys to Substantial Compliance in Cross-Border Mergers and Acquisitions
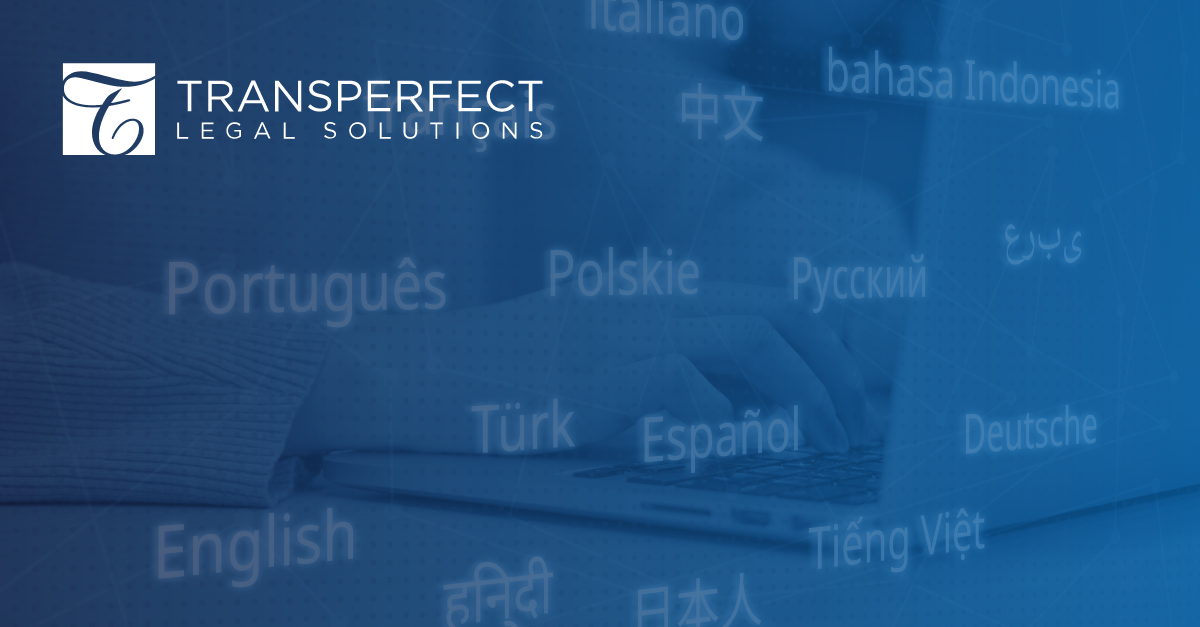
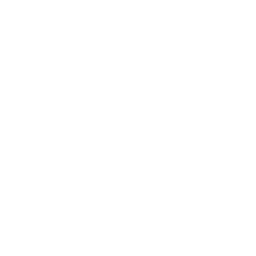
Cross-border M&A deals reached an all-time high of $2.1 trillion in 2021. Many of these transactions were subjected to merger clearance proceedings in the US and/or abroad. As a result, an unprecedented number of US second requests, Canadian SIRs, and EC Phase II proceedings involved large volumes of documents in different languages. In the big data era, multilingual data sets are not just a nuisance; they’re a serious threat to substantial compliance given the expedited schedule of most merger review proceedings. With the right workflows and technology, however, the challenge presented by large, multilingual data sets can be surmounted without breaking the bank or jeopardizing compliance. This article reviews some of the relevant tradecraft.
1. Search Term Construction
Incorrectly running search terms in a foreign language is a damaging error because poorly translated search terms result in a document universe that is both under-inclusive (relevant documents are missed) and over-inclusive (large numbers of non-relevant documents are promoted to the document review pool with all the attendant review costs). There are three common ways translated terms miss their mark.
First, because search term lists are words standing in isolation, stripped of context, it is imperative to contextualize the translation process by ensuring the linguist understands the underlying dispute, the relevant industry, and any regional dialects of the documents’ authors. By way of example, let’s look at the word “close.” One can “close” a deal, be physically “close,” be intimately “close,” “close” a door, and, in the UK, live on a “Close.” In most languages, each version of close will translate to wholly different words. In Spanish, for instance, it can become cerca, íntimo, similar, or cerrar.
Second, search term translations often miss their target when they fail to reflect the natural language expression and conjugation required in the target foreign language. Keeping with the “close” example, even if cerrar captures the right meaning of “close,” the translation will be wrong if it does not properly conjugate the verb, which is easier said than done as there are 30 separate conjugated forms of this particular Spanish verb.
Finally, syntax errors in search term translation are often the source of mis-targeting. E-discovery professionals spend years learning correct search operator usage, but linguists rarely know the art of search operators. It is paramount that a search syntax expert collaborates closely with the linguist analyzing word-by-word building in the syntax. This is especially important with respect to privilege screens due to the potential waiver implications of under-inclusive search results.
2. Multilingual Technology-Assisted Review (TAR)
To successfully manage a technology-assisted review (TAR), a.k.a. predictive coding, workflow against a multi-language data set, it is paramount to integrate two components into the workflow: (a) robust language identification tools and (b) federated machine learning models.
A. Robust Language Identification Tools
Language identification tools automatically identify the languages within each document. This includes where a single document contains more than one language—such as where the author intentionally switches languages or the body is in one language, but other information, such as a signature block, contains elements of another language. These tools also identify the percentile breakdown of the languages within each document.
Running language identification prior to commencing the TAR workflow is essential to having an informed basis on which to design the remainder of the multilingual TAR protocol. However, since language identification platforms range in accuracy (with the high-end ones approaching approximately 85%), it is also important to utilize the “right” language identification tool for your project—for example, using a tool particularly adept at CJK languages for a Korean project and a tool strong with Latin languages for a Spanish project.
Running language identification across the target data set provides an approximation of the volume of documents for each language in scope. Those volumes, in turn, dictate whether a certain minimum threshold of documents is present in a particular language to justify the use of TAR, as opposed to simply running a linear, manual review of those documents. This is particularly true in merger review proceedings because when the scope of the inquiry is the impact on global markets, the presence of small sets of certain foreign languages that do not warrant a full-blown TAR process is likely.
B. Federated Machine Learning Models
Federated machine learning models means that for each language that is in-scope for the TAR project, a separate “training set” of documents is required to train separate TAR engines or “models” for each such language—an English model for English-language documents, a Japanese model for Japanese language documents, etc. This is true regardless of whether TAR 1.0 (simple active learning) or TAR 2.0 (continuous active learning) is used. Although, in the merger clearance context, TAR 1.0 is far more common. Each training set will consist of roughly 1,500 documents that will need to be reviewed by subject-matter experts who speak the native language.
After the various training sets are complete, the resulting models are combined to create one omnibus TAR model that will guide the remainder of the project. For a TAR 1.0 workflow, the omnibus model will be used to create a control set of randomly selected documents that are used to create a benchmark of precision, recall, F1, etc. For a TAR 2.0 workflow, the omnibus model is used to assign relevance scores to all the documents, which are then used to batch to reviewers the documents most likely to be relevant. The TAR 2.0 review batches will also be filtered by the prevalent languages of the documents and the language skills of each individual reviewer.
3. Enhanced Machine Translation (MT)
Compounding the burden of managing large, multilingual datasets is the typical reluctance of antitrust and competition regulators to accept machine translations (MT) of foreign-language productions. In the United States, the Department of Justice and Federal Trade Commission will only accept MT that satisfies a standard of accuracy that “off-the-shelf” MT systems do not meet, especially given the specialized nature of the subject matter in merger clearance proceedings. In the absence of acceptable MT results, the regulators require human translations of all responsive, foreign-language documents, which is not only cost-prohibitive but also nearly impossible to execute within substantial compliance timeframes.
Fortunately, advances in the machine learning algorithms that underlie some MT platforms have given rise to enhanced MT workflows. Enhanced MT materially increases the quality of MT and more reasonably strikes a balance between the need to meaningfully review documents relating to the transaction and the costs and burdens of substantial compliance.
Similar to TAR, machine translation at its core is a prediction mechanism that is based on training. Simply put, the engine predicts the most likely translation based on the data it was trained with. Many aspects of the training process can be optimized to a particular data set with the right expertise.
There are two main MT enhancement workflows focused on the creation of task-specific training data: back translation and segment correction. Under these workflows, rather than using a generally trained, off-the-shelf engine, one or both of these data augmentation techniques may be employed to further train the engine using a custom data set for the project. The resulting content-customized engine materially increases the MT quality to a level that satisfies regulatory expectations without breaking the bank.
Of the two techniques, back translation training is faster and less costly, but the tradeoff is that it yields a more modest improvement in engine performance (up to 10% over an off-the-shelf MT engine). Segment correction, on the other hand, is more costly and time-consuming than back translation (albeit far less costly than human translation), but will result in up to 30% increased accuracy over a purely off-the-shelf MT engine.
With either approach to enhanced MT, it is important to reflect on what “quality” means. In this instance, quality means MT that more adequately carries the message from the source document and makes more appropriate use of industry-specific terminology, while maintaining a high level of fluency. Quality builds trust in MT, so when producing high quality MT, regulators will likely request fewer human translations. This quickens their review of the deal while lowering overall costs.