Four Benefits of Technology-Assisted Review 2.0: Changing and Advancing Document Review
Four Benefits of Technology-Assisted Review 2.0: Changing and Advancing Document Review
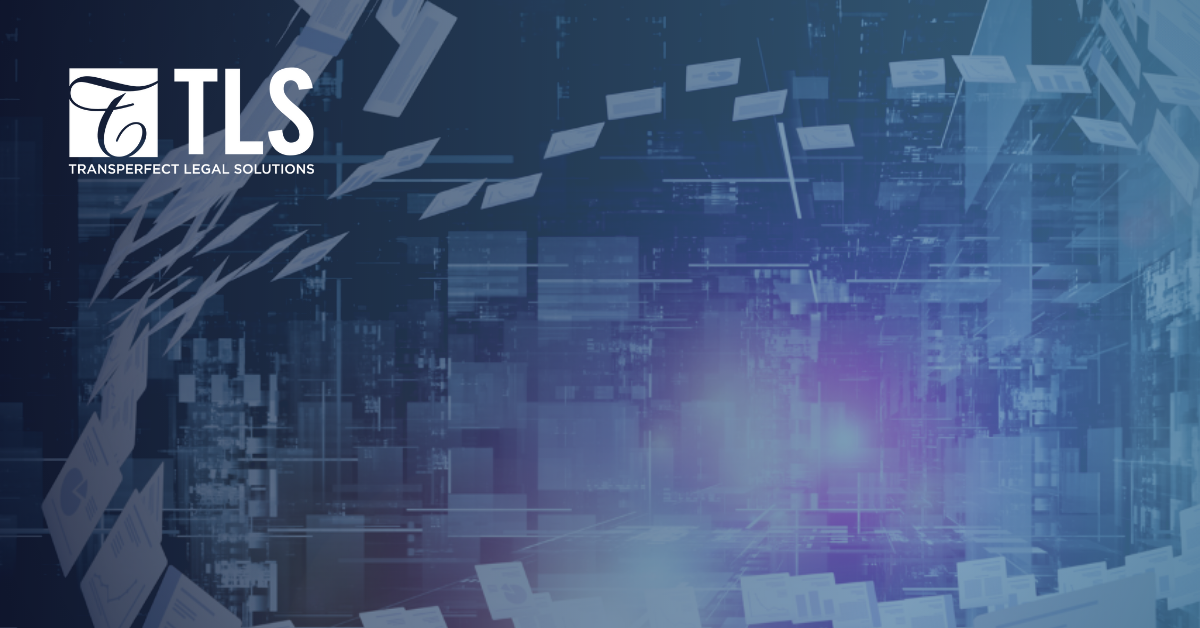
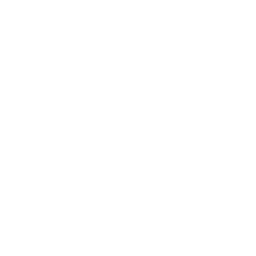
As analytic workflows become more sophisticated, the question is no longer when should your case team use technology-assisted review (TAR) – but rather, when shouldn’t your case team use TAR.
More frequently, case teams are opting to incorporate a TAR workflow to their default best practices for document review.
This shift is made possible largely due to the invention of TAR 2.0, which is also known as continuous active learning, or CAL.
How TAR Works
Instead of coding a small subset of documents and applying that learning to the larger corpus of data, as is done in a traditional TAR 1.0 workflow, TAR 2.0 is a prioritization workflow in which reviewers often put human eyes on all relevant documents.
A small initial sample set of documents is coded and then the TAR engine ranks the remaining documents based on what it has learned from the coded set, the documents with the highest ranks being most likely to be responsive.
The reviewers then concentrate on the highest ranked documents, re-ranking periodically based on newly completed coding and reprioritizing the reviewers’ document set.
This iterative process continues until the point of diminishing returns is met and the team is confident they have captured the majority of the relevant documents.
Elusion tests are conducted on the unreviewed population to ensure that the rate of elusion meets the desired goals of the review and to validate the process.
The Benefits
TAR 2.0 has gained popularity and is being embraced across the industry in a way that TAR 1.0 never quite could. Here are four ways that TAR 2.0 changed the game.
Drastically reduced the minimum document count threshold.
TAR 1.0 requires a minimum of about 50,000 documents in the data set for its value to be gained.
Because TAR 2.0 is a prioritization tool, it is valuable on data sets as small as 500 documents to organize the review and make the reviewers go faster. This allows it to be an attractive technology to use on all cases.
Eliminated the need for subject matter experts (SMEs) to conduct the review.
While the number of documents being reviewed by a human under TAR 1.0 is significantly smaller, that review should ideally be conducted by a single SME.
That SME is often a senior associate whose bill rate is expensive, and having a single person responsible can create a bottleneck in the process. This requirement made TAR 1.0 challenging if an SME was not available for the review.
TAR 2.0 learns from all coding decisions and—since the entire relevant population is reviewed—there are enough examples that a few inconsistent decisions won’t throw off the entire model, and QC methods can easily identify these outliers. This means that the review can be conducted by multiple first-level reviewers at much lower billing rates.
Allowed for full review of documents during first-pass review, including privilege and issues coding.
When conducting a TAR 1.0 review, at the end of the TAR process there is an identified set of responsive documents and an identified set of non-responsive documents, but the responsive set still needs an additional evaluation to conduct privilege review and to code for issues.
In TAR 2.0, the reviewers can also mark documents for privilege and issues as they complete the first-pass TAR review so when they reach the cutoff point, the review is complete.
An added benefit to this full review is that reviewers will identify any documents incorrectly categorized as relevant by the model and will mark them as such, a drastic improvement over the low precision typical of TAR 1.0 models where a large number of irrelevant documents are swept up into the production population in favor of high recall.
Having high precision is particularly important when potentially sensitive data is present in the document set.
Diminished the complexity of metrics needed to validate results.
TAR 1.0 relies on metrics, such as precision and recall, to validate results and to guide when the model is trained. These metrics can be difficult to understand and often require input from an analytics expert or data scientist to defend the TAR model’s success.
With TAR 2.0, it is a very simple concept for case teams to understand that when there are no more relevant documents, or very few depending on the level of risk on the case, the review is complete.
This process may still need guidance for determining the cutoff point and validating the results, but overall it is much simpler to understand and many lawyers are more comfortable with the idea that all relevant documents have been reviewed by a human reviewer.
In conclusion, TAR 2.0 has been widely adopted as the preferred method for document review because it eliminates barriers to entry and has become more user friendly.
Cases that utilize a TAR 2.0 workflow often see an average reduction of 40–60 percent in the amount of documents that need to be reviewed.
With data volumes constantly growing, using TAR 2.0 workflows will become even more important to keep document review efficient and cost-effective.
To ask questions or learn more about technology-assisted review, visit www.transperfectlegal.com/services/technology-assisted-review or contact TLS at legal@transperfect.com.